For BMS, BUS, Industrial, Instrumentation Cable.
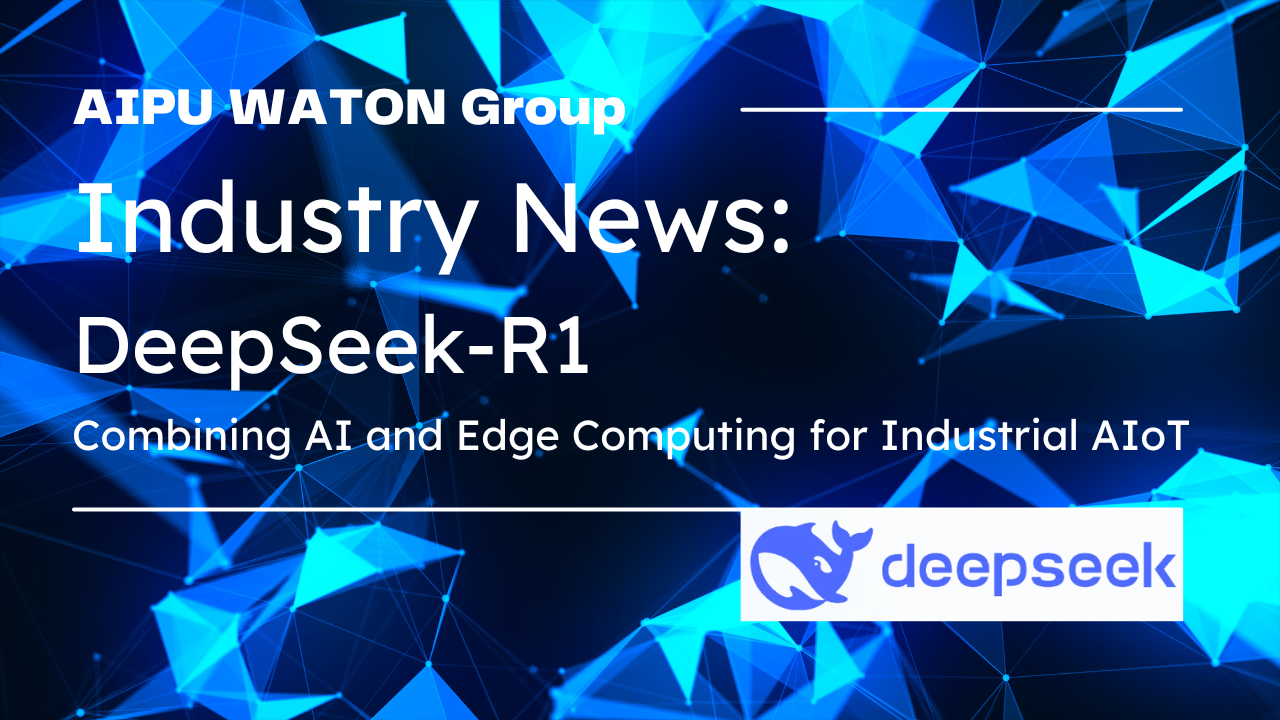
As the Spring Festival comes to a close, the excitement surrounding DeepSeek remains strong. The recent holiday highlighted a significant sense of competition within the tech industry, with many discussing and analyzing this "catfish." Silicon Valley is experiencing an unprecedented sense of crisis: advocates of open-source are voicing their opinions again, and even OpenAI is reevaluating whether its closed-source strategy was the best choice. The new paradigm of lower computational costs has triggered a chain reaction among chip giants like Nvidia, leading to record single-day market value losses in U.S. stock market history, while government agencies are investigating the compliance of the chips used by DeepSeek. Amid mixed reviews of DeepSeek overseas, domestically, it is experiencing extraordinary growth. After the launch of the R1 model, the associated app has seen a surge in traffic, indicating that growth in application sectors will drive the overall AI ecosystem forward. The positive aspect is that DeepSeek will broaden application possibilities, suggesting that relying on ChatGPT will not be as expensive in the future. This shift has been reflected in OpenAI's recent activities, including the provision of a reasoning model called o3-mini to free users in response to DeepSeek R1, as well as subsequent upgrades that made the thought chain of o3-mini public. Many overseas users expressed gratitude to DeepSeek for these developments, although this thought chain serves as a summary.
Optimistically, it is evident that DeepSeek is unifying domestic players. With its focus on reducing training costs, various upstream chip manufacturers, intermediate cloud providers, and numerous startups are actively joining the ecosystem, enhancing cost efficiency for using the DeepSeek model. According to DeepSeek's papers, the complete training of the V3 model requires only 2.788 million H800 GPU hours, and the training process is highly stable. The MoE (Mixture of Experts) architecture is crucial for reducing pre-training costs by a factor of ten compared to Llama 3 with 405 billion parameters. Currently, V3 is the first publicly recognized model demonstrating such high sparsity in MoE. Additionally, the MLA (Multi Layer Attention) works synergistically, particularly in reasoning aspects. "The sparser the MoE, the larger the batch size needed during reasoning to fully utilize computational power, with the size of the KVCache being the key limiting factor; the MLA significantly reduces KVCache size," noted a researcher from Chuanjing Technology in an analysis for AI Technology Review. Overall, DeepSeek's success lies in the combination of various technologies, not just a single one. Industry insiders praise the DeepSeek team's engineering capabilities, noting their excellence in parallel training and operator optimization, achieving groundbreaking results by refining every detail. DeepSeek's open-source approach further fuels the overall development of large models, and it is anticipated that if similar models expand into images, videos, and more, this will significantly stimulate demand across the industry.
Opportunities for Third-Party Reasoning Services
Data indicates that since its release, DeepSeek has accrued 22.15 million daily active users (DAU) within just 21 days, achieving 41.6% of ChatGPT's user base and surpassing 16.95 million daily active users of Doubao, thus becoming the fastest-growing application globally, topping the Apple App Store in 157 countries/regions. However, while users flocked in droves, cyber hackers have been relentlessly attacking the DeepSeek app, causing significant strain on its servers. Industry analysts believe this is partially due to DeepSeek deploying cards for training while lacking sufficient computational power for reasoning. An industry insider informed AI Technology Review, "The frequent server issues can be resolved easily by charging fees or financing to purchase more machines; ultimately, it depends on DeepSeek's decisions." This presents a trade-off in focusing on technology versus productization. DeepSeek has largely relied on quantum quantization for self-sustenance, having received little external funding, resulting in relatively low cash flow pressure and a purer technological environment. Currently, in light of the aforementioned problems, some users are urging DeepSeek on social media to elevate usage thresholds or introduce paid features to enhance user comfort. Additionally, developers have begun utilizing the official API or third-party APIs for optimization. However, DeepSeek's open platform recently announced, "Current server resources are scarce, and API service recharges have been suspended.”
This undoubtedly opens more opportunities for third-party vendors in the AI infrastructure sector. Recently, numerous domestic and international cloud giants have launched DeepSeek's model APIs—overseas giants Microsoft and Amazon were among the first to join at the end of January. The domestic leader, Huawei Cloud, made the first move, releasing DeepSeek R1 and V3 reasoning services in collaboration with Silicon-based Flow on February 1. Reports from AI Technology Review indicate that Silicon-based Flow's services have seen an influx of users, effectively "crashing" the platform. The big three tech companies—BAT (Baidu, Alibaba, Tencent) and ByteDance—also issued low-cost, limited-time offers starting February 3, reminiscent of last year's cloud vendor price wars ignited by DeepSeek's V2 model launch, where DeepSeek began to be dubbed the "price butcher." The frantic actions of cloud vendors echo the earlier strong ties between Microsoft Azure and OpenAI, where in 2019, Microsoft made a substantial $1 billion investment in OpenAI and reaped benefits after ChatGPT's launch in 2023. However, this close relationship started to fray after Meta open-sourced Llama, allowing other vendors outside the Microsoft Azure ecosystem to compete with their large models. In this instance, DeepSeek has not only surpassed ChatGPT in terms of product heat but has also introduced open-source models following the o1 release, similar to the excitement surrounding Llama's revival of GPT-3.
In reality, cloud providers are also positioning themselves as traffic gateways for AI applications, meaning that deepening ties with developers translates to preemptive advantages. Reports indicate that Baidu Smart Cloud had over 15,000 customers utilizing the DeepSeek model via the Qianfan platform on the model's launch day. Additionally, several smaller firms are offering solutions, including Silicon-based Flow, Luchen Technology, Chuanjing Technology, and various AI Infra providers that have launched support for DeepSeek models. AI Technology Review has learned that current optimization opportunities for localized deployments of DeepSeek primarily exist in two areas: one is optimizing for the sparsity characteristics of the MoE model using a mixed reasoning approach to deploy the 671 billion parameter MoE model locally while utilizing hybrid GPU/CPU inference. Additionally, the optimization of MLA is vital. However, DeepSeek's two models still face some challenges in deployment optimization. "Due to the model's size and numerous parameters, optimization is indeed complex, particularly for local deployments where achieving an optimal balance between performance and cost will be challenging," stated a researcher from Chuanjing Technology. The most significant hurdle lies in overcoming memory capacity limits. "We adopt a heterogeneous collaboration approach to fully utilize CPUs and other computational resources, placing only the non-shared parts of the sparse MoE matrix on CPU/DRAM for processing using high-performance CPU operators, while the dense portions stay on the GPU," he further explained. Reports indicate that Chuanjing's open-source framework KTransformers primarily injects various strategies and operators into the original Transformers implementation through a template, significantly enhancing inference speed using methods like CUDAGraph. DeepSeek has created opportunities for these startups, as growth benefits are becoming apparent; many firms have reported noticeable customer growth after launching the DeepSeek API, receiving inquiries from previous clients looking for optimizations. Industry insiders have noted, "In the past, somewhat established client groups were often locked into the standardized services of larger companies, tightly bound by their cost advantages due to scale. However, after completing the deployment of DeepSeek-R1/V3 before the Spring Festival, we suddenly received cooperation requests from several well-known clients, and even previously dormant clients initiated contact to introduce our DeepSeek services." Currently, it appears that DeepSeek is making model inference performance increasingly critical, and with broader adoption of large models, this will continue to influence development in the AI Infra industry significantly. If a DeepSeek-level model could be deployed locally at a low cost, it would greatly aid government and enterprise digital transformation efforts. However, challenges persist, as some clients may hold high expectations regarding large model capabilities, making it more apparent that balancing performance and cost becomes vital in practical deployment.
To evaluate whether DeepSeek is better than ChatGPT, it's essential to understand their key differences, strengths, and use cases. Here's a comprehensive comparison:
Feature/Aspect | DeepSeek | ChatGPT |
---|---|---|
Ownership | Developed by a Chinese company | Developed by OpenAI |
Source Model | Open-source | Proprietary |
Cost | Free to use; cheaper API access options | Subscription or pay-per-use pricing |
Customization | Highly customizable, allowing users to tweak and build upon it | Limited customization available |
Performance in Specific Tasks | Excels in certain areas like data analytics and information retrieval | Versatile with strong performance in creative writing and conversational tasks |
Language Support | Strong focus on Chinese language and culture | Broad language support but U.S.-centric |
Training Cost | Lower training costs, optimized for efficiency | Higher training costs, requiring substantial computational resources |
Response Variation | May offer different responses, possibly influenced by geopolitical context | Consistent answers based on training data |
Target Audience | Aimed at developers and researchers wanting flexibility | Aimed at general users looking for conversational capabilities |
Use Cases | More efficient for code generation and quick tasks | Ideal for generating text, answering queries, and engaging in dialogue |
A Critical Perspective on "Disrupting Nvidia"
At present, aside from Huawei, several domestic chip manufacturers like Moore Threads, Muxi, Biran Technology, and Tianxu Zhixin are also adapting to DeepSeek's two models. A chip manufacturer told AI Technology Review, "DeepSeek's structure demonstrates innovation, yet it remains an LLM. Our adaptation to DeepSeek is primarily focused on reasoning applications, making technical implementation fairly straightforward and quick." However, the MoE approach requires higher demands in terms of storage and distribution, coupled with ensuring compatibility when deploying with domestic chips, presenting numerous engineering challenges that need resolution during adaptation. "Currently, domestic computational power does not match Nvidia in usability and stability, requiring original factory participation for software environment setup, troubleshooting, and foundational performance optimization," an industry practitioner said based on practical experience. Simultaneously, "Due to the large parameter scale of DeepSeek R1, domestic computational power necessitates more nodes for parallelization. Additionally, the domestic hardware specifications are still somewhat behind; for instance, the Huawei 910B currently cannot support the FP8 inference introduced by DeepSeek." One of the highlights of the DeepSeek V3 model is the introduction of an FP8 mixed precision training framework, which has been validated effectively on an extremely large model, marking a significant achievement. Previously, major players like Microsoft and Nvidia suggested related work, but doubts linger within the industry regarding feasibility. It is understood that compared to INT8, FP8's primary advantage is that post-training quantization can achieve nearly lossless precision while significantly enhancing inference speed. When comparing to FP16, FP8 can realize up to two times acceleration on Nvidia's H20 and over 1.5 times acceleration on the H100. Notably, as discussions surrounding the trend of domestic computational power plus domestic models gain momentum, speculation about whether Nvidia could be disrupted, and whether the CUDA moat could be bypassed, is becoming increasingly prevalent. One undeniable fact is that DeepSeek has indeed caused a substantial drop in Nvidia's market value, but this shift raises questions regarding Nvidia's high-end computational power integrity. Previously accepted narratives regarding capital-driven computational accumulation are being challenged, yet it remains difficult for Nvidia to be fully replaced in training scenarios. Analysis of DeepSeek's deep usage of CUDA shows that flexibility—such as using SM for communication or directly manipulating network cards—is not feasible for regular GPUs to accommodate. Industry viewpoints emphasize that Nvidia's moat encompasses the entire CUDA ecosystem rather than just CUDA itself, and the PTX (Parallel Thread Execution) instructions that DeepSeek employs are still part of the CUDA ecosystem. "In the short term, Nvidia's computational power cannot be bypassed—this is especially clear in training; however, deploying domestic cards for reasoning will be relatively easier, so progress will likely be quicker. The adaptation of domestic cards primarily focuses on inference; no one has yet managed to train a model of DeepSeek's performance on domestic cards at scale," an industry analyst remarked to AI Technology Review. Overall, from an inference standpoint, the circumstances are encouraging for domestic large model chips. The opportunities for domestic chip manufacturers within the realm of inference are more evident due to training's excessively high requirements, which hinder entry. Analysts contend that simply harnessing domestic inference cards suffices; if necessary, acquiring an additional machine is feasible, whereas training models poses unique challenges—managing an increased number of machines can become burdensome, and higher error rates can negatively impact training outcomes. Training also has specific cluster scale requirements, while the demands on clusters for inference are not as stringent, thus easing the GPU requirements. Currently, the performance of Nvidia's single H20 card does not surpass that of Huawei or Cambrian; its strength lies in clustering. Based on the overall impact on the computational power market, the founder of Luchen Technology, You Yang, noted in an interview with AI Technology Review, "DeepSeek may temporarily undermine the establishment and rental of ultra-large training computational clusters. In the long run, by significantly reducing the costs associated with large model training, reasoning, and applications, market demand is likely to surge. Subsequent iterations of AI based on this will therefore continually drive sustained demand in the computational power market." Additionally, "DeepSeek's heightened demand for reasoning and fine-tuning services is more compatible with the domestic computational landscape, where local capacities are relatively weak, helping to mitigate waste from idle resources post-cluster establishment; this creates viable opportunities for manufacturers across different levels of the domestic computational ecosystem." Luchen Technology has collaborated with Huawei Cloud to launch the DeepSeek R1 series reasoning APIs and cloud imaging services based on domestic computational power. You Yang expressed optimism about the future: "DeepSeek instills confidence in domestically produced solutions, encouraging greater enthusiasm and investment in domestic computational capabilities going forward."
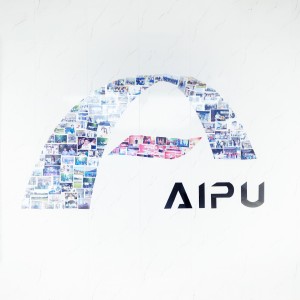
Conclusion
Whether DeepSeek is "better" than ChatGPT depends on the specific needs and objectives of the user. For tasks needing flexibility, low cost, and customization, DeepSeek may be superior. For creative writing, general inquiry, and user-friendly conversational interfaces, ChatGPT may take the lead. Each tool serves different purposes, so the choice will greatly depend on the context in which they are used.
Control Cables
Structured Cabling System
Network&Data, Fiber-Optic Cable, Patch Cord, Modules, Faceplate
Apr.16th-18th, 2024 Middle-East-Energy in Dubai
Apr.16th-18th, 2024 Securika in Moscow
May.9th, 2024 NEW PRODUCTS & TECHNOLOGIES LAUNCH EVENT in Shanghai
Oct.22nd-25th, 2024 SECURITY CHINA in Beijing
Nov.19-20, 2024 CONNECTED WORLD KSA
Post time: Feb-10-2025